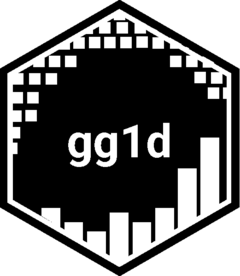
Advanced Interactivity
Source:vignettes/articles/advanced_interactivity.Rmd
advanced_interactivity.Rmd
This vignette demonstrates how to enhance the interactivity of gg1d visualizations by adding custom tooltips or cross-linking them with other plots, such as UMAP visualizations.
Adding custom tooltips
Custom tooltips make your visualizations more engaging and
informative. To add tooltips, create a new column with the
_tooltip
suffix that contains the desired text.
For example, lets add custom-tooltips to the Magpie
column of the lazy_birdwatcher dataset by creating a
Magpies_tooltip
column. Values should represent what text
to show in the tooltip.
# No Custom Tooltip
gg1d(lazy_birdwatcher)
We create a Magpies_tooltip
character column and set the
value to either "More than 3 magpies!'
or
"How boring. Too few magpies (nMagpies)"
. The gg1d function
automatically recognises this column (thanks to the suffix) and sets the
tooltip accordingly.
Cross-linking gg1d plots with other visualisations
Cross-linking enables coordinated exploration of gg1d plots with
other visualizations, produced by custom scripts or other packages. This
workflow demonstrates how to combine interactive plots using
ggiraph
and patchwork
.
As an example, we’ll cross-link a gg1d visualisation and a UMAP
visualisation summarising the palmer_penguins
dataset.
Workflow
Create your gg1d plot. Ensure you set
col_id
to embed a data-identifier required for cross-linking plots (in our case, we will use the unique name given to the penguins). Also setinteractive = FALSE
to force gg1 to returns a ggplot object instead of a htmlwidget (we’ll add the interactivity back in after we compose all our plots). For example, letsCreate the ggplot you want to co-explore with, adding latent interactivity using the ggiraph package (e.g. use
geom_point_interactive
instead ofgeom_point
and add adata_id
aesthetic set to the same column as col_id)Compose the two ggplots together using patchwork
Make the plots interactive using the ggiraph package
Example Code
library(uwot)
library(ggplot2)
library(patchwork)
library(ggiraph)
# Prepare Data & Colour Schemes
penguins <- na.omit(read.csv(system.file("penguins.csv", package = "gg1d")))
palette_species <- c(Chinstrap = "#C55BCC", Adelie = "#FF7F02", Gentoo = "#047476")
# Create gg1d plot
gg1d_penguins <- gg1d(
penguins,
col_id = "name",
interactive = FALSE,
col_sort = "species",
palettes = list(species = palette_species),
options = gg1d_options(relative_height_numeric = 1.2, show_legend = FALSE)
)
# Perform the umap
umap_mx <- umap(penguins, scale = "scale", n_neighbors = 70)
df_umap <- as.data.frame(umap_mx)
colnames(df_umap) <- c("UMAP_1", "UMAP_2")
df_umap[["name"]] <- penguins[["name"]]
df_umap[["species"]] <- penguins[["species"]]
# Create the umap plot
gg_umap <- ggplot(df_umap, aes(x = UMAP_1, y = UMAP_2, colour = species, data_id = name)) +
geom_point_interactive() +
scale_color_manual(values = palette_species) +
theme_bw()
# Combine the gg1d plot and the umap using patchwork
combined_plots <- free(gg_umap, type = "label") / free(gg1d_penguins, side = "tb")
# Make interactive
interactive_multiplot <- girafe(ggobj = combined_plots, height_svg = 6, width_svg = 10, options)
# Add some settings to choose how to make combined plots interactive
interactive_multiplot <- girafe_options(
x = interactive_multiplot,
opts_selection(type = "multiple", only_shiny = FALSE, css = "opacity: 1"),
opts_selection_inv(css = "opacity: 0.12")
)
# Draw Interactive Multiplot
interactive_multiplot